Applications of artificial intelligence in cardiovascular imaging
Research into artificial intelligence (AI) has made tremendous progress over the past decade. In particular, the AI-powered analysis of images and signals has reached human-level performance in many applications owing to the efficiency of modern machine learning methods, in particular deep learning using convolutional neural networks. Research into the application of AI to medical imaging is now very active, especially in the field of cardiovascular imaging because of the challenges associated with acquiring and analysing images of this dynamic organ. In this Review, we discuss the clinical questions in cardiovascular imaging that AI can be used to address and the principal methodological AI approaches that have been developed to solve the related image analysis problems. Some approaches are purely data-driven and rely mainly on statistical associations, whereas others integrate anatomical and physiological information through additional statistical, geometric and biophysical models of the human heart. In a structured manner, we provide representative examples of each of these approaches, with particular attention to the underlying computational imaging challenges. Finally, we discuss the remaining limitations of AI approaches in cardiovascular imaging (such as generalizability and explainability) and how they can be overcome.
Key points
- Artificial intelligence (AI) algorithms have shown impressive results in specific and often time-consuming cardiovascular imaging tasks such as image segmentation, anomaly detection and patient selection; however, these applications are limited to specific tasks in the clinical workflow.
- In cardiovascular imaging, AI algorithms are often purely data-driven but can be improved when associated with biophysical models of the heart, which enables the integration of pre-existing knowledge of human anatomy and physiology.
- A bottleneck in AI applications often lies in the collection of imaging data and their annotation by experts, which is limited by the lack of resources and expertise; therefore, the creation of large databases must be a community effort.
- The appropriate integration of AI algorithms into clinical workflows remains an unresolved problem; important security, privacy and explainability issues must be resolved to achieve a sufficiently high level of trust.
- AI algorithms have the potential to enrich the amount and the robustness of information extracted from cardiac images, while at the same time redistributing physician time and work towards patient interaction and complex decision-making tasks.
This is a preview of subscription content, access via your institution
Access options
Access Nature and 54 other Nature Portfolio journals
Get Nature+, our best-value online-access subscription
cancel any time
Subscribe to this journal
Receive 12 print issues and online access
206,07 € per year
only 17,17 € per issue
Buy this article
- Purchase on SpringerLink
- Instant access to full article PDF
Prices may be subject to local taxes which are calculated during checkout
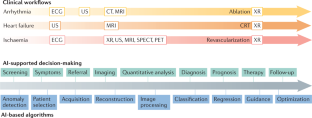
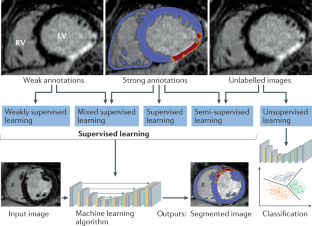
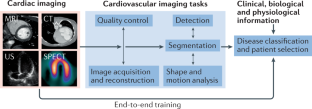
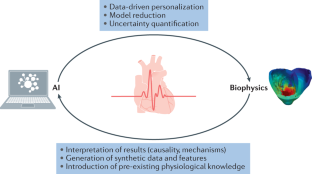
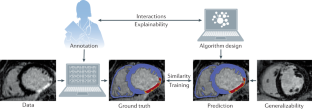
Similar content being viewed by others
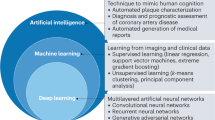
Roadmap on the use of artificial intelligence for imaging of vulnerable atherosclerotic plaque in coronary arteries
Article 18 July 2023
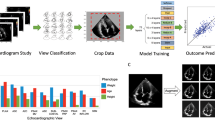
Deep learning interpretation of echocardiograms
Article Open access 24 January 2020
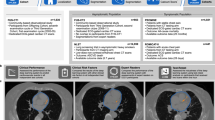
Deep convolutional neural networks to predict cardiovascular risk from computed tomography
Article Open access 29 January 2021
References
- Greenspan, H., van Ginneken, B. & Summers, R. M. Guest editorial deep learning in medical imaging: overview and future promise of an exciting new technique. IEEE Trans. Med. Imaging35, 1153–1159 (2016). ArticleGoogle Scholar
- LeCun, Y., Bengio, Y. & Hinton, G. Deep learning. Nature521, 436–444 (2015). ArticleCASPubMedGoogle Scholar
- Dey, D. et al. Artificial intelligence in cardiovascular imaging. J. Am. Coll. Cardiol.73, 1317–1335 (2019). ArticlePubMedPubMed CentralGoogle Scholar
- Siegersma, K. R. et al. Artificial intelligence in cardiovascular imaging: state of the art and implications for the imaging cardiologist. Neth. Heart J.27, 403–413 (2019). ArticleCASPubMedPubMed CentralGoogle Scholar
- Henglin, M. et al. Machine learning approaches in cardiovascular imaging. Circ. Cardiovasc. Imaging10, e005614 (2017). ArticlePubMedPubMed CentralGoogle Scholar
- O’Regan, D. P. Putting machine learning into motion: applications in cardiovascular imaging. Clin. Radiol.75, 33–37 (2019). ArticlePubMedGoogle Scholar
- Seetharam, K., Shrestha, S. & Sengupta, P. P. Artificial intelligence in cardiovascular medicine. Curr. Treat. Options Cardiovasc. Med.21, 25 (2019). ArticlePubMedGoogle Scholar
- Litjens, G. et al. State-of-the-art deep learning in cardiovascular image analysis. JACC Cardiovasc. Imaging12, 1549–1565 (2019). ArticlePubMedGoogle Scholar
- Leiner, T. et al. Machine learning in cardiovascular magnetic resonance: basic concepts and applications. J. Cardiovasc. Magn. Reson.21, 61 (2019). ArticlePubMedPubMed CentralGoogle Scholar
- Lundervold, A. S. & Lundervold, A. An overview of deep learning in medical imaging focusing on MRI. Z. Med. Phys.29, 102–127 (2019). ArticlePubMedGoogle Scholar
- Hampe, N., Wolterink, J. M., van Velzen, S. G. M., Leiner, T. & Išgum, I. Machine learning for assessment of coronary artery disease in cardiac CT: a survey. Front. Cardiovasc. Med.6, 172 (2019). ArticlePubMedPubMed CentralGoogle Scholar
- Alsharqi, M. et al. Artificial intelligence and echocardiography. Echo Res. Pract.5, R115–R125 (2018). ArticleCASPubMedPubMed CentralGoogle Scholar
- van Sloun, R. J. G., Cohen, R. & Eldar, Y. C. Deep learning in ultrasound imaging. Proc. IEEE108, 11–29 (2020). ArticleGoogle Scholar
- Cluitmans, M. et al. Validation and opportunities of electrocardiographic imaging: from technical achievements to clinical applications. Front. Physiol.9, 1305 (2018). ArticlePubMedPubMed CentralGoogle Scholar
- Alawad, M. & Wang, L. Learning domain shift in simulated and clinical data: localizing the origin of ventricular activation from 12-lead electrocardiograms. IEEE Trans. Med. Imaging38, 1172–1184 (2019). ArticlePubMedGoogle Scholar
- Bacoyannis, T., Krebs, J., Cedilnik, N., Cochet, H. & Sermesant, M. in Functional Imaging and Modeling of the Heart Ch. 3 (eds Coudière, Y., Ozenne, V., Vigmond, E. & Zemzemi, N.) 20–28 (Springer, 2019).
- Bai, W. et al. A population-based phenome-wide association study of cardiac and aortic structure and function. Nat. Med.26, 1654–1662 (2020). ArticleCASPubMedGoogle Scholar
- Petersen, S. E., Abdulkareem, M. & Leiner, T. Artificial intelligence will transform cardiac imaging — opportunities and challenges. Front. Cardiovasc. Med.6, 169 (2019). ArticleGoogle Scholar
- Attia, Z. I. et al. Screening for cardiac contractile dysfunction using an artificial intelligence-enabled electrocardiogram. Nat. Med.25, 70–74 (2019). ArticleCASPubMedGoogle Scholar
- Bernard, O. et al. Deep learning techniques for automatic MRI cardiac multi-structures segmentation and diagnosis: is the problem solved? IEEE Trans. Med. Imaging37, 2514–2525 (2018). ArticlePubMedGoogle Scholar
- Zhang, N. et al. Deep learning for diagnosis of chronic myocardial infarction on nonenhanced cardiac cine MRI. Radiology291, 606–617 (2019). ArticlePubMedGoogle Scholar
- Bello, G. A. et al. Deep learning cardiac motion analysis for human survival prediction. Nat. Mach. Intell.1, 95–104 (2019). ArticlePubMedPubMed CentralGoogle Scholar
- Bruse, J. L. et al. in Statistical Atlases and Computational Models of the Heart. Imaging and Modelling Challenges Ch. 3 (eds Camara, O. et al.) 21–29 (Springer, 2016).
- Leonardi, B. et al. Computational modelling of the right ventricle in repaired tetralogy of Fallot: can it provide insight into patient treatment? Eur. Heart J. Cardiovasc. Imaging14, 381–386 (2013). ArticlePubMedGoogle Scholar
- Grbic, S. et al. Personalized mitral valve closure computation and uncertainty analysis from 3D echocardiography. Med. Image Anal.35, 238–249 (2017). ArticlePubMedGoogle Scholar
- European Society of Radiology. What the radiologist should know about artificial intelligence — an ESR white paper. Insights Imaging10, 44 (2019). ArticleGoogle Scholar
- James, G., Witten, D., Hastie, T. & Tibshirani, R. in An Introduction to Statistical Learning Ch. 2 26–28 (Springer, 2013).
- Hu, S.-Y. et al. Can machine learning improve patient selection for cardiac resynchronization therapy? PLoS ONE14, e0222397 (2019). ArticleCASPubMedPubMed CentralGoogle Scholar
- Hosny, A., Parmar, C., Quackenbush, J., Schwartz, L. H. & Aerts, H. J. W. L. Artificial intelligence in radiology. Nat. Rev. Cancer18, 500–510 (2018). ArticleCASPubMedPubMed CentralGoogle Scholar
- Cheplygina, V., de Bruijne, M. & Pluim, J. P. W. Not-so-supervised: a survey of semi-supervised, multi-instance, and transfer learning in medical image analysis. Med. Image Anal.54, 280–296 (2019). ArticlePubMedGoogle Scholar
- Mlynarski, P., Delingette, H., Criminisi, A. & Ayache, N. Deep learning with mixed supervision for brain tumor segmentation. J. Med. Imaging6, 034002 (2019). ArticleGoogle Scholar
- Rueckert, D. & Schnabel, J. A. Model-based and data-driven strategies in medical image computing. Proc. IEEE108, 110–124 (2020). ArticleGoogle Scholar
- Saba, L. et al. The present and future of deep learning in radiology. Eur. J. Radiol.114, 14–24 (2019). ArticlePubMedGoogle Scholar
- Litjens, G. et al. A survey on deep learning in medical image analysis. Med. Image Anal.42, 60–88 (2017). ArticlePubMedGoogle Scholar
- Ronneberger, O., Fischer, P. & Brox, T. in Medical Image Computing and Computer-Assisted Intervention — MICCAI 2015 (eds Navab, N., Hornegger, J., Wells, W. M. & Frangi, A. F.) 234–241 (Springer, 2015).
- Goodfellow, I. et al. in Advances in Neural Information Processing Systems 27 (eds Ghahramani, Z., Welling, M., Cortes, C., Lawrence, N. D. & Weinberger, K. Q.) 2672–2680 (Curran Associates, 2014).
- Kingma, D. P. & Welling, M. An introduction to variational autoencoders. Found. Trends Mach. Learn.12, 307–392 (2019). ArticleGoogle Scholar
- Pesapane, F., Codari, M. & Sardanelli, F. Artificial intelligence in medical imaging: threat or opportunity? Radiologists again at the forefront of innovation in medicine. Eur. Radiol. Exp.2, 35 (2018). ArticlePubMedPubMed CentralGoogle Scholar
- Bhuva, A. et al. A multicenter, scan-rescan, human and machine learning CMR study to test generalizability and precision in imaging biomarker analysis. Circ. Cardiovasc. Imaging12, e009214 (2019). ArticlePubMedGoogle Scholar
- Oksuz, I. et al. Automatic CNN-based detection of cardiac MR motion artefacts using k-space data augmentation and curriculum learning. Med. Image Anal.55, 136–147 (2019). ArticlePubMedPubMed CentralGoogle Scholar
- Oksuz, I. et al. in Medical Image Computing and Computer Assisted Intervention — MICCAI 2019 (eds Shen, D. et al.) 695–703 (Springer, 2019).
- Schlemper, J. et al. in Medical Image Computing and Computer Assisted Intervention — MICCAI 2019 (eds Shen, D. et al.) 57–64 (Springer, 2019).
- Hyun, C. M., Kim, H. P., Lee, S. M., Lee, S. & Seo, J. K. Deep learning for undersampled MRI reconstruction. Phys. Med. Biol.63, 135007 (2018). ArticlePubMedGoogle Scholar
- Qin, C. et al. Convolutional recurrent neural networks for dynamic MR image reconstruction. IEEE Trans. Med. Imaging38, 280–290 (2019). ArticlePubMedGoogle Scholar
- Bustin, A., Fuin, N., Botnar, R. M. & Prieto, C. From compressed-sensing to artificial intelligence-based cardiac MRI reconstruction. Front. Cardiovasc. Med.7, 17 (2020). ArticlePubMedPubMed CentralGoogle Scholar
- Oksuz, I. et al. Magnetic resonance fingerprinting using recurrent neural networks. IEEE Int. Symp. Biomed. Imaginghttps://doi.org/10.1109/ISBI.2019.8759502 (2019).
- Willemink, M. J. & Noël, P. B. The evolution of image reconstruction for CT-from filtered back projection to artificial intelligence. Eur. Radiol.29, 2185–2195 (2019). ArticlePubMedGoogle Scholar
- Green, M., Marom, E. M., Konen, E., Kiryati, N. & Mayer, A. 3-D Neural denoising for low-dose Coronary CT Angiography (CCTA). Comput. Med. Imaging Graph.70, 185–191 (2018). ArticlePubMedGoogle Scholar
- Lossau, T. et al. Motion artifact recognition and quantification in coronary CT angiography using convolutional neural networks. Med. Image Anal.52, 68–79 (2019). ArticleCASPubMedGoogle Scholar
- Zhang, L. et al. in Simulation and Synthesis in Medical Imaging (eds Tsaftaris, S. A., Gooya, A., Frangi, A. F. & Prince, J. L.) 138–145 (Springer, 2016).
- Biasiolli, L. et al. Automated localization and quality control of the aorta in cine CMR can significantly accelerate processing of the UK Biobank population data. PLoS ONE14, e0212272 (2019). ArticleCASPubMedPubMed CentralGoogle Scholar
- Tarroni, G. et al. Learning-based quality control for cardiac MR images. IEEE Trans. Med. Imaging38, 1127–1138 (2019). ArticlePubMedGoogle Scholar
- Zhang, L. et al. Automatic assessment of full left ventricular coverage in cardiac cine magnetic resonance imaging with fisher discriminative 3D CNN. IEEE Trans. Biomed. Eng.66, 1975–1986 (2018). ArticleGoogle Scholar
- Dong, J. et al. A generic quality control framework for fetal ultrasound cardiac four-chamber planes. IEEE J. Biomed. Health Inform.24, 931–942 (2019). ArticlePubMedGoogle Scholar
- Robinson, R. et al. Automated quality control in image segmentation: application to the UK Biobank cardiovascular magnetic resonance imaging study. J. Cardiovasc. Magn. Reson.21, 18 (2019). ArticlePubMedPubMed CentralGoogle Scholar
- Albà, X. et al. Automatic initialization and quality control of large-scale cardiac MRI segmentations. Med. Image Anal.43, 129–141 (2018). ArticlePubMedGoogle Scholar
- Audelan, B. & Delingette, H. in Medical Image Computing and Computer Assisted Intervention — MICCAI 2019 (eds Shen, D. et al.) 21–29 (Springer, 2019).
- Vigneault, D. M., Xie, W., Ho, C. Y., Bluemke, D. A. & Noble, J. A. Ω-Net (Omega-Net): Fully automatic, multi-view cardiac MR detection, orientation, and segmentation with deep neural networks. Med. Image Anal.48, 95–106 (2018). ArticlePubMedPubMed CentralGoogle Scholar
- Zheng, Q., Delingette, H., Duchateau, N. & Ayache, N. 3-D consistent and robust segmentation of cardiac images by deep learning with spatial propagation. IEEE Trans. Med. Imaging37, 2137–2148 (2018). ArticlePubMedGoogle Scholar
- Ambrosini, P. et al. in Medical Image Computing and Computer-Assisted Intervention — MICCAI 2017 (eds Descoteaux, M. et al) 577–585 (Springer, 2017).
- Ghorbani, A. et al. Deep learning interpretation of echocardiograms. NPJ Digital Med.3, 10 (2020). ArticleGoogle Scholar
- Ghesu, F.-C. et al. Multi-scale deep reinforcement learning for real-time 3D-landmark detection in CT scans. IEEE Trans. Pattern Anal. Mach. Intell.41, 176–189 (2019). ArticlePubMedGoogle Scholar
- Noothout, J. M. H. et al. Deep learning-based regression and classification for automatic landmark localization in medical images. IEEE Trans. Med. Imaging39, 4011–4022 (2020). ArticlePubMedGoogle Scholar
- Chen, C. et al. Deep learning for cardiac image segmentation: a review. Front. Cardiovasc. Med.7, 25 (2020). ArticleCASPubMedPubMed CentralGoogle Scholar
- Isensee, F. et al. in Statistical Atlases and Computational Models of the Heart. ACDC and MMWHS Challenges (eds Pop, M. et al.) 120–129 (Springer, 2018).
- Clough, J. R., Oksuz, I., Byrne, N., Schnabel, J. A. & King, A. P. in Information Processing in Medical Imaging (eds Chung, A. C. S., Gee, J. C., Yushkevich, P. A. & Bao, S.) 16–28 (Springer, 2019).
- Duan, J. et al. Automatic 3D Bi-ventricular segmentation of cardiac images by a shape-refined multi- task deep learning approach. IEEE Trans. Med. Imaging38, 2151–2164 (2019). ArticlePubMedPubMed CentralGoogle Scholar
- Albà, X. et al. An algorithm for the segmentation of highly abnormal hearts using a generic statistical shape model. IEEE Trans. Med. Imaging35, 845–859 (2016). ArticlePubMedGoogle Scholar
- Liao, F., Chen, X., Hu, X. & Song, S. Estimation of the volume of the left ventricle from MRI images using deep neural networks. IEEE Trans. Cybern.49, 495–504 (2019). ArticlePubMedGoogle Scholar
- Margeta, J. et al. in Statistical Atlases and Computational Models of the Heart. Imaging and Modelling Challenges (eds Camara, O. et al.) 49–56 (Springer, 2014).
- Bai, W. et al. Automated cardiovascular magnetic resonance image analysis with fully convolutional networks. J. Cardiovasc. Magn. Reson.20, 65 (2018). ArticlePubMedPubMed CentralGoogle Scholar
- Gilbert, K. et al. Independent left ventricular morphometric atlases show consistent relationships with cardiovascular risk factors: A UK biobank study. Sci. Rep.9, 1130 (2019). ArticlePubMedPubMed CentralCASGoogle Scholar
- Lee, M. C. H., Petersen, K., Pawlowski, N., Glocker, B. & Schaap, M. Tetris: template transformer networks for image segmentation with shape priors. IEEE Trans. Med. Imaging38, 2596–2606 (2019). ArticlePubMedGoogle Scholar
- Zhuang, X. et al. Evaluation of algorithms for multi-modality whole heart segmentation: an open-access grand challenge. Med. Image Anal.58, 101537 (2019). ArticlePubMedPubMed CentralGoogle Scholar
- Gilbert, A. et al. in Smart Ultrasound Imaging and Perinatal, Preterm and Paediatric Image Analysis (eds Wang, Q. et al.) 29–37 (Springer, 2019).
- Huang, X. et al. Contour tracking in echocardiographic sequences via sparse representation and dictionary learning. Med. Image Anal.18, 253–271 (2014). ArticlePubMedGoogle Scholar
- Leclerc, S. et al. Deep learning for segmentation using an open large-scale dataset in 2D echocardiography. IEEE Trans. Med. Imaging38, 2198–2210 (2019). ArticlePubMedGoogle Scholar
- Asch, F. M. et al. Automated echocardiographic quantification of left ventricular ejection fraction without volume measurements using a machine learning algorithm mimicking a human expert. Circ. Cardiovasc. Imaging12, e009303 (2019). ArticlePubMedPubMed CentralGoogle Scholar
- Andreassen, B. S., Veronesi, F., Gerard, O., Solberg, A. H. S. & Samset, E. Mitral annulus segmentation using deep learning in 3-D transesophageal echocardiography. IEEE J. Biomed. Health Inform.24, 994–1003 (2020). ArticlePubMedGoogle Scholar
- Wolterink, J. M., Leiner, T. & Išgum, I. in Graph Learning in Medical Imaging (eds Zhang, D., Zhou, L., Jie, B. & Liu, M.) 62–69 (Springer, 2019).
- Itu, L. et al. A machine-learning approach for computation of fractional flow reserve from coronary computed tomography. J. Appl. Physiol.121, 42–52 (2016). ArticlePubMedGoogle Scholar
- Yang, S. et al. Deep learning segmentation of major vessels in X-ray coronary angiography. Sci. Rep.9, 16897 (2019). ArticlePubMedPubMed CentralCASGoogle Scholar
- Duchateau, N., King, A. P. & De Craene, M. Machine learning approaches for myocardial motion and deformation analysis. Front. Cardiovasc. Med.6, 190 (2019). ArticlePubMedGoogle Scholar
- Krebs, J., Delingette, H., Mailhe, B., Ayache, N. & Mansi, T. Learning a probabilistic model for diffeomorphic registration. IEEE Trans. Med. Imaging38, 2165–2176 (2019). ArticlePubMedGoogle Scholar
- Zheng, Q., Delingette, H. & Ayache, N. Explainable cardiac pathology classification on cine MRI with motion characterization by semi-supervised learning of apparent flow. Med. Image Anal.56, 80–95 (2019). ArticlePubMedGoogle Scholar
- Yan, W., Wang, Y., van der Geest, R. J. & Tao, Q. Cine MRI analysis by deep learning of optical flow: adding the temporal dimension. Comput. Biol. Med.111, 103356 (2019). ArticlePubMedGoogle Scholar
- Parajuli, N. et al. Flow network tracking for spatiotemporal and periodic point matching: applied to cardiac motion analysis. Med. Image Anal.55, 116–135 (2019). ArticlePubMedPubMed CentralGoogle Scholar
- Lu, A. et al. in Medical Image Computing and Computer-Assisted Intervention — MICCAI 2017 (eds Descoteaux, M. et al.) 323–331 (Springer, 2017).
- Song, S. et al. Deep motion tracking from multiview angiographic image sequences for synchronization of cardiac phases. Phys. Med. Biol.64, 025018 (2019). ArticlePubMedGoogle Scholar
- Attar, R. et al. Quantitative CMR population imaging on 20,000 subjects of the UK Biobank imaging study: LV/RV quantification pipeline and its evaluation. Med. Image Anal.56, 26–42 (2019). ArticlePubMedGoogle Scholar
- Mantilla, J. J. et al. Discriminative dictionary learning for local LV wall motion classification in cardiac MRI. Expert. Syst. Appl.129, 286–295 (2019). ArticleGoogle Scholar
- Duchateau, N., De Craene, M., Piella, G. & Frangi, A. F. Constrained manifold learning for the characterization of pathological deviations from normality. Med. Image Anal.16, 1532–1549 (2012). ArticlePubMedGoogle Scholar
- Sengupta, P. P. et al. Cognitive machine-learning algorithm for cardiac imaging: a pilot study for differentiating constrictive pericarditis from restrictive cardiomyopathy. Circ. Cardiovasc. Imaging9, e004330 (2016). ArticlePubMedPubMed CentralGoogle Scholar
- Sanchez-Martinez, S. et al. Characterization of myocardial motion patterns by unsupervised multiple kernel learning. Med. Image Anal.35, 70–82 (2017). ArticlePubMedGoogle Scholar
- Meyer, H. V. et al. Genetic and functional insights into the fractal structure of the heart. Nature584, 589–594 (2020). ArticleCASPubMedPubMed CentralGoogle Scholar
- Zreik, M. et al. Deep learning analysis of coronary arteries in cardiac CT angiography for detection of patients requiring invasive coronary angiography. IEEE Trans. Med. Imaging36, 1545–1557 (2019). Google Scholar
- Martin, S. S. et al. Evaluation of a deep learning-based automated CT coronary artery calcium scoring algorithm. JACC Cardiovasc. Imaging13, 524–526 (2019). ArticlePubMedGoogle Scholar
- Cikes, M. et al. Machine learning-based phenogrouping in heart failure to identify responders to cardiac resynchronization therapy. Eur. J. Heart Fail.21, 74–85 (2019). ArticlePubMedGoogle Scholar
- Alis, D., Guler, A., Yergin, M. & Asmakutlu, O. Assessment of ventricular tachyarrhythmia in patients with hypertrophic cardiomyopathy with machine learning-based texture analysis of late gadolinium enhancement cardiac MRI. Diagn. Interv. Imaging101, 137–146 (2019). ArticlePubMedGoogle Scholar
- Hilbert, A. et al. Data-efficient deep learning of radiological image data for outcome prediction after endovascular treatment of patients with acute ischemic stroke. Comput. Biol. Med.115, 103516 (2019). ArticleCASPubMedGoogle Scholar
- Bruse, J. L. et al. Detecting clinically meaningful shape clusters in medical image data: metrics analysis for hierarchical clustering applied to healthy and pathological aortic arches. IEEE Trans. Biomed. Eng.64, 2373–2383 (2017). ArticlePubMedGoogle Scholar
- Hunter, P. The virtual physiological human: the physiome project aims to develop reproducible, multiscale models for clinical practice. IEEE Pulse7, 36–42 (2016). ArticlePubMedGoogle Scholar
- Ayache, N. Medical imaging informatics: towards a personalized computational patient. Yearb. Med. Inform.25 (Suppl. 1), S8–S9 (2016). Google Scholar
- Bassingthwaighte, J., Hunter, P. & Noble, D. The cardiac physiome: perspectives for the future. Exp. Physiol.94, 597–605 (2009). ArticleCASPubMedGoogle Scholar
- Chapelle, D., Le Tallec, P., Moireau, P. & Sorine, M. Energy-preserving muscle tissue model: formulation and compatible discretizations. Int. J. Mult. Comp. Eng.10, 189–211 (2012). ArticleGoogle Scholar
- Suinesiaputra, A., McCulloch, A. D., Nash, M. P., Pontre, B. & Young, A. A. Cardiac image modelling: Breadth and depth in heart disease. Med. Image Anal.33, 38–43 (2016). ArticlePubMedPubMed CentralGoogle Scholar
- Niederer, S. A., Lumens, J. & Trayanova, N. A. Computational models in cardiology. Nat. Rev. Cardiol.16, 100–111 (2019). ArticleCASPubMedPubMed CentralGoogle Scholar
- Comaniciu, D., Engel, K., Georgescu, B. & Mansi, T. Shaping the future through innovations: from medical imaging to precision medicine. Med. Image Anal.33, 19–26 (2016). ArticlePubMedGoogle Scholar
- Molléro, R. et al. Multifidelity-CMA: a multifidelity approach for efficient personalisation of 3D cardiac electromechanical models. Biomech. Model. Mechanobiol.17, 285–300 (2018). ArticlePubMedGoogle Scholar
- Corral-Acero, J. et al. The ‘Digital Twin’ to enable the vision of precision cardiology. Eur. Heart J.41, 4556–4564 (2020). ArticlePubMedPubMed CentralGoogle Scholar
- Chabiniok, R. et al. Multiphysics and multiscale modelling, data-model fusion and integration of organ physiology in the clinic: ventricular cardiac mechanics. Interface Focus.6, 20150083 (2016). ArticlePubMedPubMed CentralGoogle Scholar
- Sermesant, M. et al. Toward patient-specific myocardial models of the heart. Heart Fail. Clin.4, 289–301 (2008). ArticlePubMedGoogle Scholar
- This, A., Morales, H. G., Bonnefous, O., Fernández, M. A. & Gerbeau, J.-F. A pipeline for image based intracardiac CFD modeling and application to the evaluation of the PISA method. Comput. Methods Appl. Mech. Eng.358, 112627 (2020). ArticleGoogle Scholar
- Vignon-Clementel, I. E., Marsden, A. L. & Feinstein, J. A. A primer on computational simulation in congenital heart disease for the clinician. Prog. Pediatr. Cardiol.30, 3–13 (2010). ArticleGoogle Scholar
- Sermesant, M. et al. Patient-specific electromechanical models of the heart for the prediction of pacing acute effects in CRT: a preliminary clinical validation. Med. Image Anal.16, 201–215 (2012). ArticleCASPubMedGoogle Scholar
- Chen, Z. et al. Biophysical modeling predicts ventricular tachycardia inducibility and circuit morphology: a combined clinical validation and computer modeling approach. J. Cardiovasc. Electrophysiol.27, 851–860 (2016). ArticleCASPubMedGoogle Scholar
- Baillargeon, B., Rebelo, N., Fox, D. D., Taylor, R. L. & Kuhl, E. The living heart project: a robust and integrative simulator for human heart function. Eur. J. Mech. A Solids48, 38–47 (2014). ArticlePubMedPubMed CentralGoogle Scholar
- Kayvanpour, E. et al. Towards personalized cardiology: multi-scale modeling of the failing heart. PLoS ONE10, e0134869 (2015). ArticlePubMedPubMed CentralCASGoogle Scholar
- Zhang, F. et al. Towards patient-specific modeling of mitral valve repair: 3D transesophageal echocardiography-derived parameter estimation. Med. Image Anal.35, 599–609 (2017). ArticlePubMedGoogle Scholar
- Lluch, È. et al. Breaking the state of the heart: meshless model for cardiac mechanics. Biomech. Model. Mechanobiol.18, 1549–1561 (2019). ArticlePubMedGoogle Scholar
- Garny, A., Noble, D. & Kohl, P. Dimensionality in cardiac modelling. Prog. Biophys. Mol. Biol.87, 47–66 (2005). ArticlePubMedGoogle Scholar
- Neumann, D. et al. A self-taught artificial agent for multi-physics computational model personalization. Med. Image Anal.34, 52–64 (2016). ArticlePubMedGoogle Scholar
- Lozoya, R. C. et al. Model-based feature augmentation for cardiac ablation target learning from images. IEEE Trans. Biomed. Eng.66, 30–40 (2018). ArticlePubMedGoogle Scholar
- Alber, M. et al. Integrating machine learning and multiscale modeling-perspectives, challenges, and opportunities in the biological, biomedical, and behavioral sciences. NPJ Digital Med.2, 115 (2019). ArticleGoogle Scholar
- Prakosa, A. et al. Generation of synthetic but visually realistic time series of cardiac images combining a biophysical model and clinical images. IEEE Trans. Med. Imaging32, 99–109 (2013). ArticlePubMedGoogle Scholar
- Duchateau, N., Sermesant, M., Delingette, H. & Ayache, N. Model-based generation of large databases of cardiac images: synthesis of pathological cine MR sequences from real healthy cases. IEEE Trans. Med. Imaging37, 755–766 (2018). ArticlePubMedGoogle Scholar
- Heimann, T., Mountney, P., John, M. & Ionasec, R. Real-time ultrasound transducer localization in fluoroscopy images by transfer learning from synthetic training data. Med. Image Anal.18, 1320–1328 (2014). ArticlePubMedGoogle Scholar
- Kissas, G. et al. Machine learning in cardiovascular flows modeling: predicting arterial blood pressure from non-invasive 4D flow MRI data using physics-informed neural networks. Comput. Methods Appl. Mech. Eng.358, 112623 (2020). ArticleGoogle Scholar
- Ayed, I., Cedilnik, N., Gallinari, P. & Sermesant, M. in Functional Imaging and Modeling of the Heart (eds Coudière, Y., Ozenne, V., Vigmond, E. & Zemzemi, N.) 55–63 (Springer, 2019).
- Coenen, A. et al. Diagnostic accuracy of a machine-learning approach to coronary computed tomographic angiography-based fractional flow reserve: result from the MACHINE consortium. Circ. Cardiovasc. Imaging11, e007217 (2018). ArticlePubMedGoogle Scholar
- Papademetris, X., Sinusas, A. J., Dione, D. P. & Duncan, J. S. Estimation of 3D left ventricular deformation from echocardiography. Med. Image Anal.5, 17–28 (2001). ArticleCASPubMedGoogle Scholar
- Finsberg, H. et al. Computational quantification of patient-specific changes in ventricular dynamics associated with pulmonary hypertension. Am. J. Physiol. Heart Circ. Physiol.317, H1363–H1375 (2019). ArticleCASPubMedPubMed CentralGoogle Scholar
- Giffard-Roisin, S. et al. Transfer learning from simulations on a reference anatomy for ECGI in personalized cardiac resynchronization therapy. IEEE Trans. Biomed. Eng.66, 343–353 (2019). ArticlePubMedGoogle Scholar
- Meister, F. et al. Deep learning acceleration of total Lagrangian explicit dynamics for soft tissue mechanics. Comput. Methods Appl. Mech. Eng.358, 112628 (2020). ArticleGoogle Scholar
- Konukoglu, E. et al. Efficient probabilistic model personalization integrating uncertainty on data and parameters: application to eikonal-diffusion models in cardiac electrophysiology. Prog. Biophys. Mol. Biol.107, 134–146 (2011). ArticlePubMedGoogle Scholar
- The Medical Futurist. FDA approvals for smart algorithms in medicine in one giant infographic. Medical Futuristhttps://medicalfuturist.com/fda-approvals-for-algorithms-in-medicine (2019).
- Saltybaeva, N., Schmidt, B., Wimmer, A., Flohr, T. & Alkadhi, H. Precise and automatic patient positioning in computed tomography: avatar modeling of the patient surface using a 3-dimensional camera. Invest. Radiol.53, 641–646 (2018). ArticlePubMedGoogle Scholar
- Taylor, C. A., Fonte, T. A. & Min, J. K. Computational fluid dynamics applied to cardiac computed tomography for noninvasive quantification of fractional flow reserve: scientific basis. J. Am. Coll. Cardiol.61, 2233–2241 (2013). ArticlePubMedGoogle Scholar
- Lu, M. T. et al. Noninvasive FFR derived from coronary CT angiography: management and outcomes in the PROMISE trial. JACC Cardiovasc. Imaging10, 1350–1358 (2017). ArticlePubMedPubMed CentralGoogle Scholar
- Bluemke, D. A. Radiology in 2018: are you working with AI or being replaced by AI? Radiology287, 365–366 (2018). ArticlePubMedGoogle Scholar
- Willemink, M. J. et al. Photon-counting CT: technical principles and clinical prospects. Radiology289, 293–312 (2018). ArticlePubMedGoogle Scholar
- Weese, J. & Lorenz, C. Four challenges in medical image analysis from an industrial perspective. Med. Image Anal.33, 44–49 (2016). ArticlePubMedGoogle Scholar
- Hutter, F., Kotthoff, L. & Vanschoren, J. (eds) Automated Machine Learning: Methods, Systems, Challenges (Springer, 2019).
- Minter, S. et al. Crowdsourcing consensus: proposal of a novel method for assessing accuracy in echocardiography interpretation. Int. J. Cardiovasc. Imaging34, 1725–1730 (2018). ArticlePubMedGoogle Scholar
- Pace, D. F. et al. Interactive whole-heart segmentation in congenital heart disease. Med. Image Comput. Comput. Assist. Interv.9351, 80–88 (2015). PubMedPubMed CentralGoogle Scholar
- Chen, L. et al. Self-supervised learning for medical image analysis using image context restoration. Med. Image Anal.58, 101539 (2019). ArticlePubMedGoogle Scholar
- Arafati, A. et al. Artificial intelligence in pediatric and adult congenital cardiac MRI: an unmet clinical need. Cardiovasc. Diagn. Ther.9 (Suppl. 2), S310–S325 (2019). ArticlePubMedPubMed CentralGoogle Scholar
- Chartsias, A. et al. Disentangled representation learning in cardiac image analysis. Med. Image Anal.58, 101535 (2019). ArticlePubMedPubMed CentralGoogle Scholar
- Sengupta, P. P. et al. Proposed requirements for cardiovascular imaging-related machine learning evaluation (PRIME): A checklist: reviewed by the American College of Cardiology Healthcare Innovation Council. JACC Cardiovasc. Imaging13, 2017–2035 (2020). ArticlePubMedPubMed CentralGoogle Scholar
- Barredo Arrieta, A. et al. Explainable artificial intelligence (XAI): concepts, taxonomies, opportunities and challenges toward responsible AI. Inf. Fusion.58, 82–115 (2020). ArticleGoogle Scholar
- Cabitza, F., Rasoini, R. & Gensini, G. F. Unintended consequences of machine learning in medicine. JAMA318, 517–518 (2017). ArticlePubMedGoogle Scholar
- European Commission. Ethics guidelines for trustworthy AI. European Commissionhttps://ec.europa.eu/digital-single-market/en/news/ethics-guidelines-trustworthy-ai (2019).
- Recht, M. P. et al. Integrating artificial intelligence into the clinical practice of radiology: challenges and recommendations. Eur. Radiol.30, 3576–3584 (2020). ArticlePubMedGoogle Scholar
Acknowledgements
Part of the authors’ work has been supported by the French Government, through the National Research Agency (ANR): 3IA Côte d’Azur (ANR-19-P3IA-0002), IHU Liryc (ANR-10-IAHU-04) and Equipex MUSIC (ANR-11-EQPX-0030). The research leading to these results has also received European funding from the ERC starting grant ECSTATIC (715093).